Digital twins are essentially dynamic, up-to-date models of a physical object or system. Centralizing data in one place eliminates data silos as all historical data and real-time data sit together. Digital twins offer a road to continuous improvement across lifecycles including better collaboration, informed decision making, reduced development time, accelerated risk assessment, optimized processes and predictive maintenance capabilities.
The digital models are fed with real data acquired by sensors within the real process. This data is processed using artificial intelligence (AI). Via the digital twins, users can simulate behaviors and predict how objects and processes will perform.
Applications for digital twins include predicting operations of products and processes in manufacturing, simulating behaviors and preventing failures in automotive production, and replicating the flow of people and raw materials to improve logistics. Digital twins are also pivotal to decreasing complexity in IoT ecosystems, according to Gartner.
“The rise of digital twins coincides with the rise of IoT. When buying machines and other assets, support for digital twins and continuous development of twin capabilities should be a selection factor,” says W Roy Schulte, Distinguished Vice President Analyst, Gartner.
Digital twins: a source of continuous intelligence
Although digital twins can be used for various applications and hold varying degrees of data, they all follow the same concept.
The digital twin is twinned with one object or process, and as a mirror image of its counterpart in the physical world, it is unique and continuously updated. When you combine the data with prescriptive analytics or optimization algorithms, the digital twin can support human or automated decision making. The digital twin can, for example, estimate the next maintenance schedule for a production line to avoid unnecessary downtime. With a digital twin, technicians can monitor wind turbines, follow their models in real time, and assess breakdown risks using machine-learning algorithms instead of going onsite, for example. This is a huge advantage in monitoring off-shore windfarms.
At Mobile World Congress this year, Orange demonstrated how a digital twin platform and an IoT application can be optimized to increase manufacturing flows. Using a digital twin together with a smart mobile tracking application, all the parts on a production line are sequenced in order of use. Any missing parts are highlighted and can be located rapidly in real time to increase the reliability of production processes while reducing costs. It also has the potential to improve the quality and speed of delivery of manufactured goods.
Digital twins deployed alongside other technologies including IoT, extended reality, data analysis and machine learning are rapidly being adopted in many industries. In France, for example, engineering company Cervval, France Énergies Marines (the French institute for energy transition dedicated to offshore renewable energies) and IMT Atlantique engineering school have launched the SubSEE 4D project to improve the operation of wind farms using a solution that couples a digital twin with deep learning methods.
In the area of health, the European Commission is funding the SimCardioTest, designed to speed up the adoption of digital simulation for the design of cardia drugs and devices.
Challenges of adopting digital twins
Creating an end-to-end digital twin platform is not an easy task. It is not a single technology but created through multiple technologies, including visualization, workflow and APIs to share data from multiple sources, a machine-learning framework for real-time decision making, and real-time data ingestion.
The concept of digital twins has yet to go mass market, so there aren’t any industry-ready products on the shelves. However, there are vendors offering industry-specific digital twins to use with their own proprietary platforms such as those targeted at the construction industry.
There is simulation software available that provides the framework for building digital twins. There are also subscription and consumption-based digital-twin solutions emerging. Microsoft Azure Digital Twins can be utilized to design a digital twin that represents actual IoT devices in a wider cloud solution. AWS IoT TwinMaker also provides a simplified approach to developers to create digital twins.
It is advisable to first create a minimum viable product (MVP) of a digital twin. This helps to identify best practices, avoid mistakes and learn from insight. The digital twin can then be scaled up from this.
Quality data and security should be top of mind
Digital twins utilize data from a host of remote sensors. To put in place effective digital twins, data quality and context are crucial. Enterprises must be able to filter out bad data to have confidence in recommendations made.
In addition, digital twins depend on sensors for their data, which are connected to IoT devices. Each endpoint represents a possible security vulnerability.
Attacking a digital twin could provide cybercriminals with business-critical information. Therefore, it is essential that enterprises address their security postures on deploying digital twins. This includes looking at encryption and access privileges and running security audits.
Consider working with a partner
Digital twins are proving to be invaluable across industries. As we have outlined earlier, creating digital replicas comes with challenges when it comes to issues such as data quality and security. Many enterprises may see business cases for digital twins but not have the insight to start on a project.
This is where a partner like Orange Business can help guide you through creating digital twins and ensure that you build simulations that best match your business requirements.
Orange Business experience in digital twins and IoT includes condition monitoring, predictive maintenance, automated quality control and the management of fuel, energy and water usage.
With the cost of digital-twin technologies declining, there has never been a better time to implement digital replicas to improve design and productivity efficiencies.
Learn more about how digital twins are being developed in industries like medicine, energy and transport.
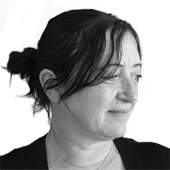
Jan has been writing about technology for over 22 years for magazines and web sites, including ComputerActive, IQ magazine and Signum. She has been a business correspondent on ComputerWorld in Sydney and covered the channel for Ziff-Davis in New York.