Training data is labeled data that is used to develop AI and machine learning models. The quality of this data has enormous implications for the success of these models and their decision-making capabilities. The higher the quality of the data, the better the models will perform overall. That is why AI practitioners spend vast amounts of time collecting high-quality data to build their models. Inaccurate, incomplete or irrelevant data sets can skew results.
In addition, if the data used for the training is not adequately diverse and unbiased, it can create AI bias issues. One of the most infamous examples was Amazon’s AI recruiting tool that showed a bias against applications from women. This is because it had been trained using resumes submitted to the company over ten years. Most resumes came from men, and the system thus taught itself that men were preferable candidates. Amazon recruiters quickly recognized the bias, and despite correcting it, the project was shelved.
“There are headwinds facing AI adoption,” explains George Livingston, Principal, Business Group at Orange Silicon Valley. “These include company cultures that don’t recognize AI, difficulties in recognizing use cases for the technology and lack of practitioners. Lack of data and data quality are big issues, plus some enterprises do not have the right infrastructure in terms of connectivity and sensors.”
A move to small and wide data
Big data is often cited as central to building efficient AI programs. Large chunks of structured and unstructured data are consistently fed to train AI. However, one of the big challenges that enterprises face, unless they are data-centric companies like Amazon or Google, is getting access to huge and reliable data sources. Designing a framework that will accommodate an extensive, scalable data program is also complex and resource hungry.
This is where so-called small data comes in. Big data tends to be concentrated in a few industries such as IT, healthcare and automotive, which constrains new use cases for AI. Small data, however, is widely available, providing an opportunity to unlock new use cases with more efficient and responsible approaches. Small data also provides a greater diversity of data than was available in the past, allowing it to be analyzed more effectually, providing fast and powerful insights.
Gartner predicts that by 2025, 70% of organizations will move the focus from big to small and wide data, providing greater context for analytics and making AI less data hungry.
“Disruptions such as the COVID-19 pandemic are causing historical data that reflects past conditions to quickly become obsolete, which is breaking many production AI and ML models,” explains Jim Hare, Distinguished Research Vice President at Gartner. “In addition, decision making by humans and AI has become more complex and demanding and overly reliant on data-hungry deep-learning approaches.”
As we advance, AI technology must operate on small data and adaptive machine learning, says Gartner. At the same time, these systems must also protect privacy, comply with regulations and minimize bias to support ethical AI.
Hare recommends that data and analytics leaders adopt both small data and wide data. Wide data is combining diverse data sets from various structured and unstructured data sources. For instance, researchers used AI to review thousands of research papers, clinical trial data, news and social media to predict the spread of COVID-19, capacity plan and find new treatments.
“Taken together, they are capable of using available data more effectively, either by reducing the required volume or by extracting more value from unstructured, diverse data sources,” Hare says.
Small data has a place in the start-up landscape
Many AI practitioners believe that small data is AI’s highway to the mainstream. Why? Because it is neither possible nor feasible to have big data available for every challenge. Start-ups are fast seeing the value of small data for many companies.
Wendy Gonzalez, CEO of start-up Sama, is passionate about providing AI with the right data diet for optimum results. The company offers high-quality training data, validation and annotation solutions for AI and ML models. It works with several major names, including NASA, Ford and Walmart.
Gonzalez believes that small data in training AI models has a vital role to play. Speaking at Orange Silicon Valley’s Hello Wednesday webinar Training data: the hidden engine behind AI, she underlines the importance of data as the foundation for all AI applications. Feeding AI a diet of junk data gets junk results. “When people evaluate their training data strategy, they need to consider quality, security and ethics. An effective training strategy factors in secure data handling, rigorous quality checks and ethical data sourcing,” she says. Sama consistently produces data with 94-98% accuracy using machine-assisted annotation combined with ethical human validation.
“Humans in the loop are necessary for context and quality, plus assist us with the machine annotation. You need incredible precision, and that last bit is the human touch to make an outline, for example, or understand the context of metadata,” adds Gonzalez.
To reduce bias, Gonzalez stresses the importance of having a platform built by a diverse population, providing diversity in the data and who is labeling it.
Juno Millin, a founder of start-up DroneDeploy, also believes that small data has a big role to play in AI training, especially in industries that have been slower to digitize, such as construction and agriculture. DroneDeploy captures professional-grade imagery using drones and analyses them to check crop yields or building safety. It also integrates images into a host of apps. It uses humans in the loop and sees itself as machine-assisted rather than fully automated. “There may be risks in the future if people make decisions from automated data,” he says.
Smaller and smarter
By deploying smarter, more responsible, scalable AI, organizations can utilize learning algorithms and interpretable systems in shorter timeframes to harvest greater value and bolster the bottom line by applying analytics for enhanced decision making.
“Small and wide data approaches provide robust analytics and AI while reducing organizations’ large data set dependency,” concludes Rita Sallam, Distinguished Research Vice President at Gartner.
Hear from AI start-ups like DroneDeploy, Sama and Intenseye by watching Orange Silicon Valley’s Hello Wednesday virtual event Training data: the hidden engine behind AI. To find out more about smarter data management for AI-enabled business success, click here.
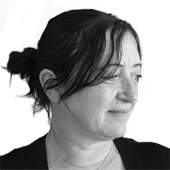
Jan has been writing about technology for over 22 years for magazines and web sites, including ComputerActive, IQ magazine and Signum. She has been a business correspondent on ComputerWorld in Sydney and covered the channel for Ziff-Davis in New York.