IDC predicts that global data creation and replication will continue unabated at an annual growth rate of 23% from now until 2025. It is paramount that enterprises capture value from this data explosion. According to Gartner, if done successfully, chief data officers (CDOs) can increase business value by a factor of 2.6.
The big issue is how to manage this data effectively and make it easily accessible. Enter data mesh, which essentially disseminates data and puts it in the hands of business domains. These specific teams own, manage and serve data up as a product to the rest of the business.
Adopting data mesh means putting data consumers at the heart of the process. Domain teams themselves are consumers and producers of new data sets at the same time, composed of data scientists, engineers and business analysts.
Data mesh: a distributed way of looking at data
Data mesh isn’t a product. It is a concept that moves away from monolithic structures, represented by data warehouses and data lakes. A data mesh describes a distributed domain-driven and self-service platform approach, where data is treated as a product.
Unlike data warehouses and data lakes, where specialist technical staff often don’t understand individual domains’ data but are responsible for it, data mesh creates “data-as-a-product.” This allows each domain to control its data pipeline and quality. Data mesh provides data that is secure and easy to access, significantly enhancing the user experience.
In addition, the domains host and serve their own data sets for which they are responsible in an easily consumable way. They are not dependent on centralized engineering teams ingesting data from all the domains into one data lake.
For data mesh to be a success, data consumers must be actively involved in the processes. Domain data owners must understand what data users require, how they are using it, and the best ways they like to consume data. This is in comparison to centralized teams, who are only responsible for the implementation of the data but will not consume it.
Data mesh requires a paradigm change in thinking
Data mesh opens up distributed data sets, providing faster access and accurate data delivery. But because data mesh is more than technology, it requires a significant change in organizational culture to succeed.
For many enterprises, this means moving to a federated governance model, built on cross-organizational trust and leveraging a data-domain-oriented self-service design. Self service simplifies data access, breaks down silos, and enables the scaled-up sharing of live data.
Domains are responsible for their data
Each individual domain is responsible for domain-related use cases or solves specific business issues. This ensures high data quality as the data processing is tasked to the teams that have the most knowledge about the use cases.
In contrast, data lakes built on a centralized approach often run into problems because engineering teams responsible for each implementation step don’t have any specific domain knowledge. In addition, data producers are often demoralized to fulfill requirements as their output does not relate to any particular use case. It also makes it difficult for consumers to get value from data.
Central components for data governance and infrastructure
Data mesh incorporates major elements for data governance and infrastructure, which work as self-service platforms to support product-owner workflows and make sure connecting parts in the infrastructure work in harmony.
A scalable, practical governance framework is central to the success of data mesh. Competent governance eliminates the technical complexities linked to managed domains while ensuring that data moves consistently across them. It also ensures compliance and data visibility at a granular level, ensuring data is secure and managing domain meta information.
Open or strict management
Data mesh provides two different approaches to managing domains: open and strict. While the open model gives domain teams as much unrestricted movement as possible, the strict model is designed to support domain teams in highly regulated environments. Hybrid approaches are also possible.
Via the open model, domains have no limitations in choosing their tools for data processing and storing. This approach, however, demands reliable and responsible domain teams to avoid any inconsistencies and variable data quality.
In the strict model, domains have no access to their infrastructure code and thus must stick to the standard set of resources. It is important to note that the strict approach requires extensive implementation and automation efforts from within the platform team and implies a highly sophisticated mesh platform.
Data mesh overview
As enterprises harvest, store and analyze more and more data, so the concept of decentralized data ownership becomes very clear. Putting data back into the hands of the people that understand it is ultimately the way forward. But creating a distributed self-service architecture with centralized governance takes ongoing commitment and is not a simple tick-box activity.
Moving from a monolith to a microservices model requires enterprise reorganization and cultural change. But this transformative paradigm is worth the effort, as it can accelerate an enterprise’s data-centric vision.
For an in-depth view of data mesh and what it can do for your enterprise, download our white paper: Introducing data mesh: A future‑proof approach to managing company‑wide data at scale. Also, check out or webinar: Data Mesh: A Paradigm Shift to Unlocking the Full Value of Data.
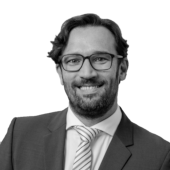
Georg Rösch is a senior engineer with The unbelievable Machine Company. His background in business informatics enables him to combine data engineering and data insights with a profound understanding of strategic business decisions based on evidence. As an engineer of *um’s “Data at Rest and Data in Move” team, he is involved in several data platform projects including conception, implementation and automation of data mesh, data lakes, data pipelines, tagging frameworks and data analytics solutions.