We can refer to Albert Einstein to guide our approach:
“Given one hour to save the world, I would spend 55 minutes defining the problem and 5 minutes finding the solution.”
How can IT help?
Thankfully, we have more than an hour to save the world. We now have a better comprehension of the non-medical problem at hand, so let’s look at the role of IT and explore the application of analytics, artificial intelligence (AI), machine learning (ML) and the Internet of Things (IoT).
IT is tasked with reducing costs and reducing risks, and its application can lead to significant benefits.
1. Supply forecast
By creating a common data store to get visibility of the demand, we can ensure that the vaccine blocs are delivered in such a manner with zero waste. This includes all aspects of the cycle being fed to the drug manufacturers and the logistics companies to ensure the right cargo size of drugs is shipped, and on time. Analytics of this data, combined with additional data points from the area, could also help accurately forecast demand. A key aspect here is data security. Appropriate security controls must be in place, for example, measures, such as encryption and hashing, to ensure prevailing data privacy and other regulatory laws are adhered to and to protect the integrity of the supply chain.
The benefits of leveraging analytics with AI give a turbo boost to the benefits, such as those detailed in this whitepaper. These benefits are all applicable to the COVID vaccine logistics despite the uniqueness of the cryogenic supply chain. There’s a pressing need in this crisis to ensure that the challenges of leveraging AI and analytics are addressed properly and in advance, so that AI delivers optimal vaccine distribution.
2. Leveraging IoT and ML, including embedded ML, computer vision and deep learning, while providing remote connectivity to all supply devices
The idea of using sensors to track aspects like temperature, GPS, number of times opened, remaining stock, etc. is probably self-evident. However, there will be some key considerations to consider, like selecting the sensor set with the right attributes, including form factor, power consumption and ability to work in harsh and varied climate conditions. Selecting and testing such sensors for their suitability in the harshest of environments is critical because they will be responsible for feeding data. If this data is wrong or interrupted, then other stages in the chain could fail.
Once we solve the challenge of the right set of sensors, the next question is connectivity: ensuring the data from the devices is transmitted. This is a complex challenge because of power consumption patterns and the varied scenarios, including indoor, outdoor and patchy cellular coverage. Each package needs multiple connectivity options, such as cellular, UWB, LoRA, BLE and RFID, according to the environment of operation and the need for redundancy. For example, UWE / BLE are better suited for indoors within warehouses, whereas cellular is better suited for in the field or road transportation. The goal is the lowest energy consumption possible, allowing the portable energy units to be focused on their primary purpose of powering refrigeration.
The sensor data only becomes valuable when used for actionable insight and predictions. Experience has taught all of us that bad news needs to be conveyed three times faster than good news to allow timely corrective action. We can apply that principle in this scenario by the application of machine learning to predict faults before they occur rather than retrospectively.
The challenging mix of poor connectivity, connectivity costs and the fact that every vaccination requires its own environment/context from manufacturing to end user, makes it sensible to embed the learning component at the edge. Machine learning for IoT typically sends all the data from the sensors to the cloud. This data is then used to train the model in the cloud. Most uses of ML on the edge are basically using this trained model and deploying it on the edge to spit out decisions on the live data. The model, however, is centrally trained with data distributed across multiple devices. By embedding the learning component onto each device, we achieve the possibility of each device being trained based on its unique data.
This machine learning model is complex and demands to be federated to be able to be trained on the edge, taking into account the environmental conditions of each individual box. The temperature of a vaccine container could change more drastically in a hot desert than on the icy plains of a steppe. Hence, each device trains its own model and, once the degree of accuracy is acceptable, then executes it. This is a form of unsupervised learning. We can also consolidate all the models and training data in batches to extract the commonalities, processing this data in areas where the connectivity is good and cheap.
Embedded ML is enabled via a software application that is purpose built for embedded systems and runs on edge devices. It typically runs on an embedded computer, therefore allowing it to consume less resources than a cloud implementation.
Embedded ML complements edge and cloud computing. They work together to enhance performance. ML performed by an embedded software application is intended to enable learning directly on devices to form more valuable data before sharing it with the central data model.
Embedded ML leverages a concept called incremental learning, as opposed to batch learning, which is used for most traditional machine-learning models.
Benefits of embedded ML include:
- Reduced amounts of data: producing data with higher value before sending it to the cloud realizes potential cost savings on bandwidth or cloud-computing resources
- Improved response time for latency-sensitive applications and cognitive ML needs
- Autonomous operation and reduced dependency on cloud/network connections
- End-user or device personalization, including local environmental parameters
All sensors do not necessarily need to be on the devices. Through a combination of strategically deployed smart cameras and analysis of the various video streams, we can further deploy computer vision and deep learning for sites, providing the ability to monitor inventory conditions as well as the workers accessing them.
3. Worker safety working with cryogenically frozen product
The risks and safety measures of handling cryogenically frozen goods must be considered. Given that most vaccines are being developed using mRNA technology, they currently require storage and transportation at < -20°C (up to -75 °C). It is important to note that this technology will also be relevant for many more vaccines to come. To ensure and monitor site and worker safety, we should ensure that adequate measures are put in place to detect exposure of personnel working with the vaccines.
As per NCNR, the top three cryogenic hazards are:
1. Cold burn, leading to frostbite and hypothermia
As per this study on wearables for continuous occupational cold stress assessment, the cold tolerance is very subjective and designing a personalized wearable sensor used in combination with ML. This may by time consuming as well as cost prohibitive.
A simpler starting point would be to have solid-state temperature sensors, which can be quickly inserted into wearables to gain insight into skin temperature. There have been some very interesting DIY projects to build such wearables, which could launch more professional production of these capabilities embedded into PPE kits. If these alerts, which would be added to audible signals to the user, can also be transmitted to a central server, then they could ensure that appropriate assistance alerting and predictive modelling are performed.
This would be critical to catching these exposures and reacting early to prevent accidents and injury.
2. Asphyxiation, due to boiling off and oxygen displacement
Cryogenic cooling generally involved using super-cooled liquids, such as carbon dioxide, helium and nitrogen. These super-cooled liquids, if accidentally leaked, will immediately boil over to their gaseous forms and will displace oxygen. This is especially dangerous in confined spaces like closed rooms, warehouses and walk-in fridges. Oxygen sensors have been around since the 1960s, thanks to Robert Bosch Gmbh and Dr. Günter Bauman.
A combination of room sensors connected to monitoring systems will ensure adequate early warning of gas leaks.
3. Explosion of sealed containers
The boiling of cryogenic liquids creates a bigger risk for sealed containers due to the phenomenon of thermal expansion. Using connected sensors to measure not just temperatures but also pressure would be critical to analyzing and preventing the containers from exploding.
In my first two blogs on the challenges of delivering vaccines to the world, I have discussed how IT can be of service in vaccine supply-chain logistics, including how even some of the most advanced techniques, such as artificial intelligence and machine learning, can assist in the drive for the most efficient vaccine rollout possible. In future blogs on this topic, I’ll deal with other major challenges, like security, and examine what role Blockchain can play in distributing this life-saving vaccine across the globe.
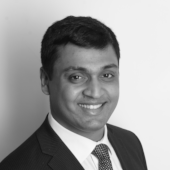
Ashwin is Head of Consulting at Orange Business, Switzerland, where he specializes in helping organizations embrace digital transformation. He is a technology enthusiast, cloud evangelist, IoT adopter, AI student and, when he has spare time, enjoys music, cricket and gastronomy.