The question of whether to use AI or not is no longer relevant. Any business that wishes to grow in the coming years will use artificial intelligence, and that’s not a hard prediction to make.
Two-thirds of enterprise tech executives are prioritizing generative AI investments, while 75% of executives say AI has already met or exceeded their expectations.
The technology’s potential is huge, while the barriers to entry are tumbling. The market is growing rapidly, as every conceivable type of vendor, from the Big Four to brand-new start-ups, roll out different tools, services and models. In 2020, just $44 million was invested in foundation models; by 2023, that had swelled to $16.3 billion.
Consequently, enterprises are faced with a wide array of choices. Yet the breadth of options available, coupled with the relative ease of access, creates a situation where simply having AI is not a competitive differentiator.
AI’s value comes from how you use it
How do you overcome this? By focusing on how you use it.
There is a danger that in the excitement surrounding AI, many businesses will buy the tech before thinking about the problem. Yet AI is just that: technology. It might offer huge opportunities and create new revenue streams no one can imagine. However, it is still a tool that needs to be integrated properly into a business to be successful.
Businesses must avoid getting carried away with the hype but instead focus first on one thing: what is the problem they are trying to solve?
It might be that you don’t have enough developers to write the code to build the apps your customers expect. It might be that you know you’ve got the right information somewhere in your organization but can’t find it. It might be that your contact center agents are spending too much time on triage and not enough on escalated queries.
Whatever it is, once you find your problem, you can start developing your use case. This will determine what AI you need and how to use it.
Scaling sustainably
Being clear on your use case will help you choose the right AI and develop the skills, frameworks and policies needed to implement the technology properly and safely.
This is vital, as AI deployments need to be able to scale. You start with one use case, something that will generate results quickly, take the learnings and build them into the next project. This helps develop a critical mass, which convinces doubters and ensures that your AI growth is sustainable.
And it must be based on real-world experience, not lab-based theories. Large language models might be built with millions of possible parameters, but there will always be something unforeseen that challenges your AI tool. It is much easier to handle if it occurs in a focused use case than if you have tried to roll out AI across the entire organization simultaneously.
Identifying unforeseen consequences
This scaled approach also allows you to bring the right parties on board, not just superusers who will constantly be exposed to the tools. This is important because every action has consequences, and deploying AI is no different.
For instance, biases must be prevented from being introduced by AI. We all have personal prejudices, and they are unconsciously built into everything we humans develop. Looking at individual use cases helps weed out potential biases before they can be amplified across much wider sections of the organization.
Strong governance and clear frameworks are also needed. For instance, the use of large language models needs to be carefully monitored due to the possibility of hallucinations.
By taking a use-case-by-use-case approach, issues such as data privacy, security and regulatory compliance can be considered within the project's parameters, making it easier to manage.
Integrating sticks and stones with rocket science
Then there is the question of integrating AI with your existing tech stack. Throughout the history of IT, integration has been inconsistent; sometimes, different solutions work well together, and often, they don’t. Even tools created by the same vendor don’t always play nicely.
In some instances, AI is like introducing rocket science to an environment used to sticks and stone. This issue must be overcome rapidly; AI’s potential will never be reached if it cannot eventually reach all parts of the organization. Any savings, efficiencies or new revenue opportunities will disappear.
A scaled use case approach allows new tooling to be carefully integrated with adjacent technology, tested and proofed before it is expanded.
It’s not what you’ve got that matters
AI is going to change the world, and part of the reason for that is that everyone can use it. However, in the rush to be part of the revolution, businesses must focus on the problems they want to solve, not the solution. Identifying use cases that will realize value rapidly not only helps generate results but also ensures that any AI deployment can be scaled sustainably.
Defining the use case is central to AI deployment success, but so is a strong understanding of what AI means for security, data and the network, and the role of LLMs. To find out more, take a look at our new ebook.
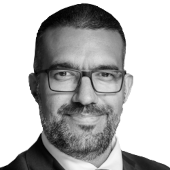
As VP Data & AI and Director of Marketing, Innovation, and Strategy for Digital Services at Orange Business, Michaël leads development projects within the group's ESN activities and is an evangelist for data, AI and generative AI.