A data lake is basically a storage method for all of an enterprise’s raw data gathered from diverse sources. It stays dormant there until a business use is identified for it. Andrew White, VP at Gartner refers to it as “a staging area for data between a number of sources and some kind of consumer/consumption.”
Data is doubling in size every two years. Data is now one of an enterprise’s most important assets, so keeping it safe and usable is crucial. This is where data lakes come in – they don’t replace data warehouses, they complement them. Data lakes are highly flexible and versatile and are best suited to analytics work. Data warehouses, on the other hand, deal with structured data, optimizing it for retrieval across an entire organization. Thus, storing all your data in data warehouses and databases can be expensive as it needs to be cleansed and prepared before storage, although source data itself isn’t retained. Scaling is expensive and requires specific hardware.
“Data lakes don’t replace anything but are a new and additional part of the data infrastructure that solves new problems. Data warehouses are still very important for companies, but not as flexible as data lake systems,” explains Ingo Steins, Deputy Director of Operations at The Unbelievable Machine Company, an Orange Business company.
Data lakes promise to pull down data silos by creating a single repository for your entire organization. They enable unstructured data and source data to be retained and stored in a cost-effective way.
Data lakes are designed for parallel processing and linear scaling. Unstructured data – ranging from social media data and doc files and pdfs to information harvested from connected devices – is pouring into enterprises. Airplane engine sensors, for example, produce 20 terabytes of data per hour. With a data lake, enterprises can inexpensively store this data, drawing on it for analysis when the need arises.
But while data lakes have proven successful for storing massive quantities of data, they only work if they are carefully managed and you know exactly what is in them. Otherwise a pristine data lake can fast turn into an information quagmire.
Keeping your data lake crystal clear
Data lakes have many benefits including flexibility, the ability to derive value from unstructured data types and unlimited ways to query data.
“That might sound like a dream. And it is,” Steins continues. “But it might also become your worst nightmare. Structure and documentation are key even for data lakes. You simply must know what’s in the lake. Otherwise, what you get is not a beautiful data lake but something more like a data swamp.”
“Poor data quality levels lead to bad results, even with a perfect analysis platform,” adds Steins.
A data lake doesn’t mean simply creating a basin and pouring your data in ad hoc. That makes it messy, unmanageable and leads to what has been dubbed a "data swamp."
Avoiding a "data swamp" scenario is paramount if an enterprise is to truly exploit and capitalize on its data and generate new business intelligence. There are simple ways, however, of keeping your data lake crystal clear.
First, be selective with your data. One of the biggest mistakes that enterprises make is to collect too much data simply because it is available. Work out what you want the data for and the business challenges it might solve. This can avoid flooding your data lake with data that will have no purpose down the line. Knowing where its priorities are is central to the development of a governance structure for any enterprise.
Data governance introduces practices to data lake management that optimize the value of the data, classify and protect it, while making it clear who owns it – be it structured on unstructured data. This ensures that the data lake holds reliable data assets and meta data than can be easily found at all times. To this end, Steins believes it is “important to define a person responsible for what is in the data and data quality in each department.”
You can also take advantage of emerging technologies, such as artificial intelligence (AI) and machine learning, to sort the data, spot patterns and pinpoint its value.
Finally, it’s beneficial to keep your data lake in close proximity to where you plan to use the data. The farther away the data lake, the more you are likely to suffer from latency issues when it comes to data analysis, for example. Keeping your data close to users shores up security and optimizes data usage, fueling increased productivity.
Data fabric – helping link the data lake
With growth in the digital economy, the inevitable is happening. Enterprises are starting to move to multiple data lakes. One example is duplicating data from one data lake to another data lake in a different geographic location. This must be done using consistent data management and governance to ensure data security, which is not an easy task. This is where data fabric steps in to provide a management layer across all the data lakes.
Data fabric is basically a mix of architecture and technology housed in a single data management platform. It has been created to overcome the complexities of managing diverse data formats using multiple database management systems that run across various platforms – including on-premises, in data centers and in multi-cloud environments.
Data fabric solutions, such as Splunk, create a scalable unified data environment, woven into the very fabric of an enterprise’s information systems. They support multiple locations, improving central data flow coordination, services and reliability.
Moving forward
With our increasingly connected world, data challenges are increasing. Unifying data generated from a growing number of applications is becoming more and more problematic. Enterprises need to bring together data from data lakes, data warehouses, cloud storage and so forth and make sure they can turn it into a business asset – not a burden.
Data fabric might just be the answer to the data dilemma that enterprises are facing, avoiding "data swamps" in not one, but a multi-data lake environment.
For more, read our ebook on maximizing value with data.
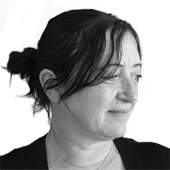
Jan has been writing about technology for over 22 years for magazines and web sites, including ComputerActive, IQ magazine and Signum. She has been a business correspondent on ComputerWorld in Sydney and covered the channel for Ziff-Davis in New York.