ML is a subset of artificial intelligence (AI) that enables machines to build problem-solving models by determining patterns in data and spotting anomalies. “The learning refers to the training process – the algorithms identify patterns to tweak the model, aiming to provide a more accurate output each time,” explains Gartner. ML can be broadly categorized into two categories – supervised learning, where ML learns from training data, and unsupervised learning, where it finds associations in datasets.
Attacks are growing: 83% of organizations studied by the Ponemon Institute last year had suffered more than one data breach. Reaching an all-time high, the cost of a data breach averaged $4.35 million in 2022.
ML can help address the growing number of cybersecurity challenges by detecting attacks at all levels, including hard-to-detect polymorphic viruses that are programmed to mutate, such as Cryptowall ransomware and WannaCry.
Increased connections increase exposure
The threat landscape is continually expanding thanks to an accelerating number of endpoints, including IoT and remote working devices. Add to this a shortage of cybersecurity professionals, and it is clear that security teams are always playing catchup. ML is a game changer in building a strong security posture in a dynamic environment.
ML augments human experience and skills while safeguarding against human error. It can identify and profile devices on the network, automatically find any anomalies, provide insight at scale and detect zero-day attacks. The latter are previously unknown threats that often target unprotected vulnerabilities.
ML algorithms can be deployed within network traffic analysis to detect network-based attacks, such as a distributed denial of service (DDoS) attack, where online services are brought to a standstill by flooding the system with synthetically generated traffic. ML can strengthen endpoint protection and shore up anti-virus and firewall solutions by rapidly identifying unknown threats. ML is also used in fingerprint and facial recognition software and provides authentication to stop account takeover.
In addition, ML can be used to gain security insights across an entire complex infrastructure. Orange Cyberdefense, for example, used ML to analyze World Watch advisories in its Security Navigator 2023 report, designed to provide insights for a proactive security posture.
Orange Cyberdefense used natural language processing (NLP), a subset of machine learning (ML), to automate several aspects of data classification, entity extraction and scale analysis alongside experts.
Overall, ML enables enterprises to automate repetitive tasks, improve technology models and develop an evolving and responsive line of defense against malicious actors.
Criminal use
However, it is important to understand that cybercriminals are also using ML. When it comes to phishing, for example, which relies on human failure to launch an attack, ML is used to train AI to create scenarios comparable to real life. This enables them to work out where they will get the best results. AI and ML are also used to seek out vulnerabilities to exploit and create more sophisticated malware.
Criminals will also look to disrupt enterprise ML-based security to gain an advantage. To protect yourself, it is vital to have real-time visibility across your network and the ability to add context and spot anomalies.
ML comes with challenges
ML brings considerable benefits in advancing cybersecurity tools, but it isn’t perfect. ML is only as smart as the people and practices used to deploy it. It could actually weaken defenses if it isn’t learning from the correct data by mismarking traffic as safe, for example.
Data plays a pivotal role in the machine learning process. One of the main challenges the success of ML faces is the lack of good-quality data. “Dirty” data can lead to inaccurate or faulty predictions. The issue is that much security data is classified as sensitive and not freely available. In addition, getting adequate malware samples while it is continually evolving is difficult.
Enterprises need a highly integrated approach to ML and data harvesting, organizing and modeling to work effectively. This is no small task but brings with it great rewards.
Ground truth can also be troublesome in ML, as it deals with a progressive landscape. Ground truth is the data known to be true, provided by direct observation. It provides a gold standard on which to evaluate results. With malicious actors continually developing sophisticated attack tools, it is impossible to have a permanent ground truth.
Gaining insights
Orange Cyberdefense realized ML’s limitations and possible drawbacks in its analysis. ML models can yield results that, if taken at face value, could mislead or result in false claims. This meant carefully considering the False Positive rate or the True Negative rate. There can also be hidden bias in models that can frame the results.
Orange Cyberdefense noted, however, that “the correct usage of ML can lead to gains in productivity and scale that would never have been possible before. This means we can respond quickly to new information and make better decisions.”
Extracting real-time, high-quality insights is central to the success of ML in cybersecurity. Unfortunately, a huge skills gap and talent shortage in data science is even more acute in ML and cybersecurity. It is also no secret that for an enterprise to build and deploy its ML tools supported by ML specialists is a costly exercise.
ML will greatly impact cybersecurity in the coming years, helping enterprises protect their assets and users. But it needs expertise, organized data streams and continuous investment to live up to expectations.
To find out more about how Orange Business can help you use ML to give meaning to your data, click here.
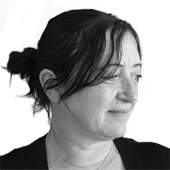
Jan has been writing about technology for over 22 years for magazines and web sites, including ComputerActive, IQ magazine and Signum. She has been a business correspondent on ComputerWorld in Sydney and covered the channel for Ziff-Davis in New York.